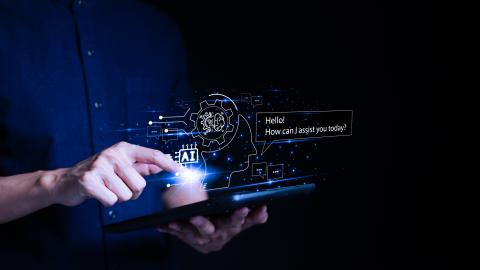
Generative AI: The algorithms revolutionising content creation
This article was originally published in L'Édition n°25.
Since the launch of ChatGPT in 2022, generative artificial intelligence (AI) has continually found new applications in various areas of our day-to-day lives. But what is generative AI? How does it work? And what are its benefits and limitations? We take a deeper look at a technology that has become ubiquitous.
"Over the last few decades, artificial intelligence (AI) has made a spectacular headway, and among its most promising branches is generative AI. Unlike traditional algorithms, generative AI is capable of creating new data which has never been seen before. This possibility opens up unprecedented horizons in a wide variety of fields, including copywriting..."
If you haven't had a chance to test the capabilities of generative AI yet, you've just had a demonstration. The paragraph above was not written by a human, but by ChatGPT, the chatbot from OpenAI. Launched in November 2022, the impact of this system on the general public has been revolutionary. Two months after its launch, it already had over 100 million users. ChatGPT is still the best-known and most widely used generative AI, with around 1.8 billion monthly visits to the dedicated platform.
Université Paris-Saclay has also been swept along in this generative AI revolution. But how is this reflected? This question was addressed at a day-long event organised on the Plateau de Saclay on 5 June by the Pedagogical Innovation Department (DIP) and the Graduate School for Research and higher education (GS MRES), with the support of the DATAIA Institute, Université Paris-Saclay's artificial intelligence institute. "The aim was to demystify generative AI and offer a multidisciplinary overview of the use of tools like ChatGPT at the university," explains Serge Pajak, researcher at Network, Innovation, Territories, Globalization laboratory (RITM - Univ. Paris-Saclay) and Coordinator of the Generative AI project at the university. "ChatGPT has become so popular that many students are already using it. In a way, this obliges the academic community to take a position in relation to this technology, to guide best practice." Teachers at Université Paris-Saclay have already taken up the technology, for example, by asking the chatbot questions on the course material and discussing its response with students. "It is an interesting method because it is highly effective for integrating AI into teaching and applying it in a critical way, by discussing the quality of its content."
Mapping the uses and competences of generative AI within the university is in fact Serge Pajak's main role. "With the DIP and the GS MRES, I am also working on building a support system for using these tools. Specifically, the idea is to offer training in the form of acculturation, together with a fairly practical approach, as well as raising awareness of the potential misuses of this technology." For example, misuse could be allowing ChatGPT to decide whether or not to validate a project after analysing the file. But "its internal mechanics are not at all hardwired for that," Serge Pajak asserts. To understand why, we need to delve into the very heart of generative AI, or rather of the multiple systems that fall under this term.
Generative AI: a single term for very different systems
Although people think generative AI has emerged only recently, it is the fruit of several decades of innovation in the field of machine learning. These innovations have accelerated over the last ten years, and this has given rise to generative AI as we know it. "We should emphasise first and foremost that generative AI is a very commercial term. It is a key word used as a communication engine, but it is too vague in scientific terms," warns Vincent Guigue, a lecturer attached to the Applied Mathematics and Computer Science laboratory (MIA - Univ. Paris-Saclay/AgroParisTech/INRAE).
In practice, generative AI is indeed based on a scientific concept: computer systems capable of generating data. "The problem is that we are grouping very different architectures together under this umbrella." The term is used for ChatGPT, designed to generate text, MidJourney, which is used to create images, GitHub Copilot, conceived to provide computer code, and AlphaFold, specialised in protein modelling. "From a technical perspective, these models are not at all based on the same components, nor the same data modalities," the lecturer explains.
Although their architectures differ, generative AI models share a common basis: deep learning. These are highly complex mathematical operators made up of elementary units: artificial neurons. These are grouped into networks which are made up of dozens or even hundreds of layers of interconnected neurons which analyse the data supplied. The hyper-flexible architectures can be adapted to a wide variety of data types - text, images, sound, digital data, etc. - for equally diverse applications. Natural Language Processing (NLP) is just one of these.
In artificial intelligence, "processing textual information is an extremely complicated task," explains Vincent Guigue. "There are hundreds and hundreds of thousands of words. Not to mention the spelling mistakes we make every day. Handling textual data is therefore difficult." Yet this is exactly what ChatGPT and other tools, such as Claude, developed by Anthropic, or LaMDA, created by Google, are now capable of.
Language models: "fantastic tools" for processing text
Their secret lies in language models, architectures specifically designed to process text. "These models are interesting because they can better represent textual information, by projecting words into a continuous vector space. Each word becomes a point in space." And the position of each of these points is vital. The closer the points are, the more they have a similar meaning. There are also regularities in this space: when making translations, we switch from a feminine to a masculine noun, from singular to plural, and so on. When transferred into a vector space, a text becomes easier to analyse and understand.
In fact, it is not really the words that are analysed. The models perform what is known as tokenisation: the text is divided into small elements called tokens, which are words, syllables or sequences of characters. Dividing the text up like this helps the models have less linguistic variability, adapt to spelling mistakes, or model multiple languages with a limited vocabulary. But in order to properly process the texts supplied to them, the algorithms first have to train themselves on vast amounts of data. ChatGPT, for example, is based on a Transformer model, or more precisely a GPT (Generative Pre-trained Transformer), which is trained to predict the next token. Its task is to complete a sequence, token by token, with the most likely sequence possible, based on the previous context.
This analysis capability is the basis for a wide range of applications. "Language models are fantastic tools for classifying words, extracting insights from documents or summarising texts," confirms Vincent Guigue. The reason why ChatGPT has been revolutionary is that the chatbot goes much further than that. "Up until now, these models have been intermediate tools for getting better at language processing tasks. But the fact that a model is capable of generating knowledge-based texts and giving relevant answers to complex questions, that is totally new."
Optimised output thanks to reinforcement learning
OpenAI is based on a Large Language Model (LLM). In other words, a much larger neural network trained on much more data. At its launch, the chatbot ran on a version of GPT-3 with 175 billion parameters. By way of comparison, "the transformer model in vogue in 2017 had 100 million parameters, which was already huge," recalls Vincent Guigue. As regards the volume used to train it, there is a colossal body of several terabytes (TB) of data, comprising hundreds of thousands of articles, books and other texts from the web. "It is estimated that the whole content of Wikipedia makes up just 3% of the archive used for training ChatGPT."
The model trained itself on this massive body of data to predict the next word, in order to model the language and memorise knowledge. But OpenAI hasn't stopped there. Thanks to additional, more high-quality data, the company has also trained the GPT in the question-and-answer game and other reasoning tasks. Finally, "there was a final reinforcement learning stage with a human in the loop: a question was put to the system, which generated 10 answers. The human operator then noted the answers, directing the system to the most relevant ones. Thanks to this step, the quality of our answers became much higher. We can also censor sensitive subjects," explains the lecturer. Before ChatGPT, this type of learning had never been used to optimise the output of a model.
Thanks to this specific learning process, the chatbot is now able to respond in startlingly natural language to the wide range of requests (or prompts) it receives. Whether writing a speech or finding a recipe, ChatGPT always follows the same process: identifying the most likely next word, one after the other. "For ChatGPT, the answer is a succession of plausible words that follow the sentence which was given as the input." That's why, as mentioned above, this type of system is unsuited for being asked its opinion on a file or homework assignment.
Language processing is not the only application to witness spectacular advances thanks to neural networks. This is also the case for image creation, where advances have been made in systems such as MidJourney, which can now generate images that are stunningly realistic. "The field of machine learning is not moving at the same speed as other sciences, far from it. Looking back over the past ten years, it has been a bullet train. My fellow teachers and I all had to update our courses more than five times, otherwise we would be teaching things that were no longer relevant," confirms Vincent Guigue.
Models that make mistakes
Despite the fact that generative models have become successful, they still have their limitations. ChatGPT and similar systems "have no notion of what is good or bad in their answers. They have a notion of what is likely and what is not likely," stresses Vincent Guigue. They are therefore perfectly capable of generating errors, answers that are statistically correct but factually wrong. These “hallucinations” are particularly common when the machine is asked about knowledge that is rare, or that dates from after its training data. But "the models don't know how to say they don't know. They are very confident in their correct answers as well as their mistakes."
Another essential limitation of the models is their lack of predictability and explainability. Why did this model give this answer rather than another one? "We are currently unable to explain the decision-making process of these models, because there are too many combinations of parameters." Studies have shown that certain unusual prompts, such as asking the machine to “take a deep breath”, improve the quality of its responses. Although no-one knows why.
"There are plenty of applications where the limits of the models are not acceptable. If we say a model is 97% reliable, that sounds good. But a self-driving vehicle that is 99% reliable would be a disaster," the lecturer explains, citing as an example the fatal accident in 2018 involving an Uber vehicle and a pedestrian. "The day after the accident, we knew everything about what had happened, we were able to explain everything after the fact. The problem was that we hadn't been able to predict that the algorithm would make mistakes under certain conditions."
Medicine is another field where generative AI has recently made its entry. "It is very good that doctors can benefit from assistance in diagnostics. Algorithms can highlight elements that doctors have overlooked, and which may be logical," confirms Vincent Guigue. "But these things can also be completely illogical. That's why it is essential that the decisions are still taken by humans. These systems are very good assistants, but not very good replacements."
References :
- The conference of June 5 2024 (in French) : https://parissaclay.mediasite.com/Mediasite/Channel/iagenerative/
Generative AI and ethics: the challenge of regulating an emerging technology
The rise of generative AI is not without its challenges and risks. This revolutionary technology raises many ethical and legal questions, linked to its design, uses and societal impact. Various initiatives are attempting to regulate generative AI at the global level, but they are confronted with the challenge of legislating for a technology that is still in its early stages of development.
An historic law came into force in the European Union (EU) in August 2024. Called the AI Act, it aims to lay the foundations for regulating artificial intelligence (AI) within the EU. The European bodies had been working on this draft legislation for three years, with intense debate along the way. And for good reason: at the global level, the EU is the first to adopt legislation to address the rise of AI, including generative AI.
While in a general sense AI raises various ethical and legal questions, generative AI systems prompt their own set of questions. Large language models (LLMs), like the one used by ChatGPT, are capable of generating what is known as toxic language. These include insults, hate speech, incitement to violence, offensive or disrespectful statements. Some content is also likely to reflect bias, such as racist or discriminatory stereotypes.
To reduce this type of output, the companies behind the systems are applying filters or controls via human moderators during the models' learning phase. However, this process is currently neither transparent nor verified. It is not infallible either. "These filters and controls do not eliminate toxic language. They just make it less likely," confirms Alexei Grinbaum, Director of Research at CEA Paris-Saclay and Chairman of CEA's Digital Ethics Operational Committee. Conversely, this moderation can also lead to excessive oversight.
The origin and quality of the data used to train the models is another source of concern. "What we have here is the first level of generative AI ethics. It is all about the technical aspect: how are the models designed and how are they being used?" questions the physicist from the CEA. "But we also have another type of ethical consideration regarding the long-term effects of this technology on society and human beings. For example, how are the models changing professional practice?"
Experts in digital ethics already had their concerns about the challenges of these systems before the launch of ChatGPT in November 2022. The National Pilot Committee for Digital Ethics (CNPEN), under the aegis of the National Advisory Ethics Council (CCNE) and set up two years earlier, published its first opinion on chatbots in 2021. "We discussed the Transformer language models and their impact on society. At the time, we were already anticipating the scale and magnitude of the issues that would be raised," recalls Alexei Grinbaum, member of the CNPEN and co-rapporteur of this opinion. A second opinion was published in 2023, with around thirty recommendations for designing, researching and governing generative AI systems. The CNPEN - whose mission ends in 2024, making way for a permanent body - "was the first at the national and international level to take a stand on these questions."
An "essential" regulatory framework
The challenges involved in these systems are so pressing that "it is essential to put a regulatory framework in place," Alexei Grinbaum believes. With this in mind, the European Union adopted the AI Act, the measures of which will gradually begin to apply from 2025. Among the most important provisions, this legislation introduces the notion of high-risk AI systems when used in critical domains such as education, employment or the issuing of bank loans. "This means that all systems operating in the sectors in question are subject to obligations and certifications," Alexei Grinbaum explains. Similarly, the Act establishes a classification of general-purpose AI models, with various obligations imposed according to the associated risk.
In the AI Act, "we find all the principles that already existed for AI in 2019: transparency, explainability, robustness, and security. What I find very interesting is that it establishes a new ethical principle: making distinctions." Indeed, the Act requires that content generated by a model is distinguishable from content created by a human. "This is a fundamental principle that we discussed with the CNPEN. But from a scientific perspective, it is difficult to implement for certain content." Elements such as watermarks are proving effective in helping identify AI-generated images and videos. With text, on the other hand, the task is far more complex. "There are obligations in the AI Act for which we currently have no scientific solutions," the specialist admits.
This example, among others, illustrates the challenge of ”operationalising” - putting into practice - the principles laid down in the Act. According to Alexei Grinbaum, it also underlines the importance of establishing dialogue between the technical players in the sector and the regulatory bodies. This is all the more crucial as generative AI is a rapidly emerging and evolving technology: "Eight months is the equivalent of a century in the field of artificial intelligence. The advance of these technologies is much faster than the legislative process." Is legislating AI the best solution? "The problem is that we have to adopt legislation which is flexible enough to evolve very quickly so that we don’t regret it in five or ten years' time. Which, in my opinion, is not completely the case with the AI Act." In May 2025, the AI Office, set up by the European Commission, is due to publish codes of practice to enforce the EU's AI Act. "That is when we will see the concrete, practical scope of the Act's highly political articles," predicts Alexei Grinbaum.
Elsewhere too, similar initiatives are seeing the light of day. In 2023, China, along with the U.S., introduced new regulations to govern the development and use of AI, including generative AI. In the hope of catching up with the evolutions of recent years. But the technology will probably always stay one step ahead. "With this technology spreading to all sectors, it is not possible to consider every angle. It is clear that in 20 years' time, there will be a whole host of new questions that we can't even imagine yet," Alexei Grinbaum predicts.
References :
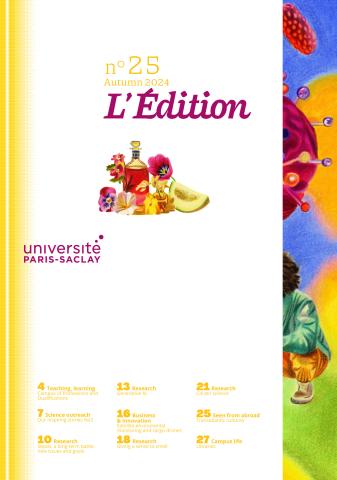
This article was originally published in L'Édition n°25.
Find out more about the journal in digital version here.
For more articles and topics, subscribe to L'Édition and receive future issues: