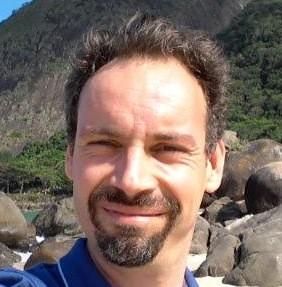
Balázs Kégl
With a PhD in computer science, Balázs Kégl has been a researcher in the Linear Accelerator Laboratory of the CNRS and the chair of the Center for Data Science of the Université Paris-Saclay since 2014.
He has published more than hundred papers on unsupervised and supervised learning, large-scale Bayesian inference and optimization, and on various applications. At his current position he has been the head of the AppStat team working on machine learning and statistical inference problems motivated by applications in high-energy particle and astroparticle physics.
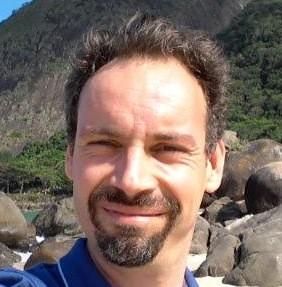
Balazs Kegl, you are the coordinator of the CDS (Center for Data Science) flagship project. What are the main goals of this project?
The Paris-Saclay Center for Data Science (PSCDS) is an interdisciplinary initiative of the recently inaugurated Université Paris-Saclay. It loosely groups about 250 scientists in 35 laboratoires. Roughly half of us are data scientist (doing research in statistics, machine learning, signal processing, data visualization, databases), and half of us are domain scientists working with data (in physics, biology, environmental sciences, social sciences, and neuroscience).
The goal of this initiative is to establish an institutionalized agora in which these scientists can find each other, exchange ideas, initiate and nurture interdisciplinary projects, and share their experience on past data science projects. To foster synergy between data analysts and data producers we propose to provide initial resources for helping collaborations to get off the ground and to mitigate the non-negligible risk taken by researchers venturing into interdisciplinary data science projects.
Besides seed-financing concrete projects, we have also been designing and learning to manage generic tools to accompany data science projects with different needs. We organize innovation and strategy workshops, coding sprints, hackatons, bootcamps, and data challenges. We see the CDS as an opportunity for experimenting with novel and unconventional forms of organizing labor and training around high-added-value projects.
Your community is very active as evidence. What is the goal of these bootcamp and data analytics sessions? Who benefits from this training?
Bootcamps or hackatons are single-day collaborative coding sessions for solving a relatively well-defined data science problem. After thorough preparation, a data provider arrives with a problem and an associated data set. We get together 20-30 participants and 3-5 coaches. We present the problem and the data for about an hour, form teams of 2-3 persons, and tackle the problem during the day. The goal of the day is to design a solution that _combines_ individual contributions while maintaining a healthy competition between the teams. We have been building both software and management tools to foster creativity, diversity, and collaboration in the room. We are very early in this process, so I can't give you more details at this point, but we are all very excited about the prospects of inventing new ways to organize work in general.
Besides the problem-solving goal, bootcamps are also training sessions. We have a wide variety of people with different expertise: statisticians with little coding expertise, software engineers with no data analysis skills, domain scientists who crunch data regularly but don't know the latest techniques, or even scientists who are novice to data science but who have a lot of data to analyze. The goal is to learn from each other by doing hands-on data science.
A third goal of the bootcamps is networking. The sheer size of Université Paris-Saclay is staggering. It gives us an unparalleled opportunity to build a data science community with a critical mass that exists in perhaps a handful of cities around the world. We have about sixty people registered for the bootcamps, and we are expecting this number to grow. The bootcamps are thus not only problem-solving sessions but also networking events that will forge social contacts between our experts.
In your opinion, what are the three most difficult issues in data science?
At the forefront of AI there are difficult technical questions: how to build systems that can adapt to their environment and communicate with us in a transparent way, how to process data streams real-time (because we can no longer store everything), how to make machines understand common sense. Nevertheless, I would say that most of the current obstacles are sociological-organizational: how to find the right experts for given problems, how to make smart people with different expertise work together efficiently while remaining collectively creative, how to balance the desire that the machine understands us with keeping sensitive data about us private.
We often talk about Big data as a scientific and economic leverage for the next 10 years. In what areas are the most significant today?
It is almost impossible to overestimate the effects of data science in the economy and in our society in the near future. Those who use IT products regularly have noticed the quantum leap in computer vision or speech recognition systems in the last couple of years, but the profound changes will come when the traditional (non-IT) industry wakes up and starts to apply powerful AI technologies in their products. There are so many things going on at the same time that it is hard to predict how our world will look like in ten years. I give you one example: self-driving cars. It is unlikely that city driving can be automatized in the next ten years, but we are not far from making highway driving autonomous. This means that most of the ~50 million truck drivers of the world will lose their jobs. And if you think your high-skill job is safer, read this. At the same time, thanks to technologies that connect supply and demand by a click, on demand work is rising, forcing classical companies to adapt or perish. We will have to learn to work less, and institutions and social tools have to adapt.
In my mind the biggest challenge today is speed. We are not prepared for the changes, and our institutions are even less well equipped than individuals. Our politico-economical elites are living in the past, and cannot solve problems for which simple solutions exist. Our children are formatted in school for a world that existed twenty or even fifty years ago. To hit closer to home: our universities will also have to adapt: both what we teach and how we teach are rapidly becoming obsolete. To finish on a positive note: Université Paris-Saclay, for the simple reason that it is something new and fresh, is a great place to be now: it's a challenge to build it, but it can easily become one of the centers where the university of the 21st century is invented.