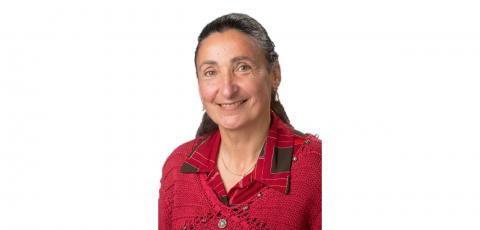
Algorithms that learn and classify: Isabelle Guyon's work recognised
The creation of computers which themselves determine how to classify data was the achievement enjoyed by Isabelle Guyon and her colleagues Bernhard Schölkopf and Vladimir Vapnik in the 1990s. This year, they were celebrated by the BBVA foundation for this work, which has already been recognised internationally as a major advancement in the field of machine learning - an area of artificial intelligence. Let’s take a look back at this work and at the career of Isabelle Guyon, now professor of Big data at Université Paris-Saclay and a member of the Tackling the Underspecified (TAU) team together with Inria Saclay and the Interdisciplinary Digital Science Laboratory (LISN - Université Paris-Saclay, CNRS, CentraleSupélec, Inria).
Programming algorithms that learn using examples is at the heart of machine learning, or automated learning. While research and applications in this field are flourishing today, they were still in their infancy in the 1990s. At this time, Isabelle Guyon was working in the Bell laboratories in the United States, where she met researchers Bernhard Boser and Vladimir Vapnik. Together, they developed a very successful data sorting technique called SVM - Support Vector Machine or wide margin classifiers.
SVM - classification algorithms, and much more besides
A SVM algorithm is an automated learning algorithm. If it is provided with data representing different categories of objects - learning examples - the algorithm is able to derive the information necessary to determine rules differentiating these categories of data. It can subsequently predict whether a new piece of data falls into any of the categories. “It was originally a classification algorithm, recalls Isabelle Guyon. But its use has since spread to other types of tasks and applications. For example, classification problems help with sorting spam or with medical diagnosis.ˮ
Cited more than 11,000 times, the original publication describing the SVM algorithm is a benchmark in the field of machine learning. “SVM’s are the culmination of several ideas - that of the wide-margin algorithm formulated by V. Vapnik in the 1960s and the Kernel methods which I studied for my PhD,” points out Isabelle Guyon. The wide-margin algorithm developed by Vapnik classifies objects according to an amount determined by the object's parameters: each parameter has a numerical coefficient and their sum (weighted by the numerical coefficients) determines the category of the object. This algorithm, which is a linear classifier, optimizes the margin between learning samples to obtain a more accurate classification of the data after learning. The Kernel methods expand the range of possibilities by classifying linearly inseparable data. The SVMs that have been developed also use terms that are still used in numerical optimization, such as error minimisation, which is the fraction of examples that are misclassified during learning. In short, these algorithms are proving to be very fruitful for the scientific community. Bernhard Schölkopf, the co-recipient of the BBVA foundation prize, has contributed to the spread of Kernel's methods following the popularity and success of SVM.
Several professional roles
After working at the Bell Labs, Isabelle Guyon set up “Coplinet” in 1996 in California. This was a machine learning consultancy. She worked from home while bringing up her children. A few years later, she returned to France. In 2016, she joined Université Paris-Saclay, where she she was given a teaching chair in Big data, then received a chair in Artificial Intelligence from the ANR. A good understanding of data and the methods available in the field of machine learning are at the heart of her teaching. “Data is essential when you're doing machine learning. So I always work with students on their understanding of data and the importance of collecting it. There are a lot of methods that can be studied. I try to make sure that students understand how these methods work because, in order to use them properly, you must not just use them blindly - you have to understand what's going on.”
The specialist in machine learning also works in the Tackling the Underspecified (TAU) team, together with Inria Saclay and the Interdisciplinary Digital Science Laboratory (LISN - Université Paris-Saclay, CNRS, CentraleSupélec, Inria). In partnership with RTE, she is developing methods based on machine learning aimed at optimising the distribution of electricity on the grid of the French electricity network.
Artificial intelligence for all
Isabelle Guyon is passionate about promoting artificial intelligence and machine learning methods to a wide audience. While she was working at Clopinet, she founded ChaLearn - a non-profit organisation which organises machine learning challenges, which she chairs. Today, ChaLearn is still is setting up challenges in partnership with local authorities such as the Île-de-France region, or with major companies such as Dassault or RTE. Students, researchers and engineers who wish to train in artificial intelligence all take part. Formulating the problem in the form of a challenge, in which hundreds of people participate, makes it possible to find the optimal solution. “I think that AI methods can be misused. However, if they are available to as many people as possible, individuals are likely to make better use of them at all levels,” points out Isabelle Guyon, adding “We would like these tools to be free, easy to use and accessible to all.”
The researcher is delighted with the award from BBVA foundation, which, in her view, also recognises the contribution of all those who have contributed to the development of machine learning.