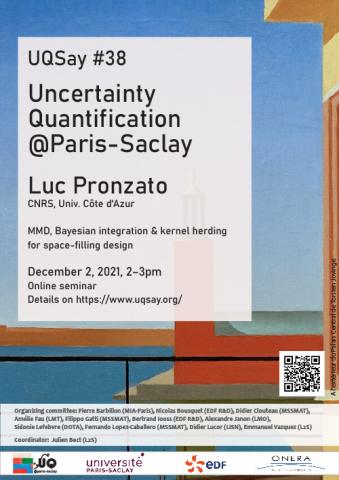
UQSay Seminar #38: Maximum Mean Discrepancy, Bayesian integration and kernel herding for space-filling design
A standard objective in computer experiments is to predict/interpolate the behaviour of an unknown function f on a compact domain from a few evaluations inside the domain. When little is known about the function, space-filling design is advisable: typically, points of evaluation spread out across the available space are obtained by minimizing a geometrical (for instance, minimax-distance) or a discrepancy criterion measuring distance to uniformity.
We focus our attention to sequential constructions where design points are added one at a time. The presentation is based on the survey], built on several recent results that show how energy functionals can be used to measure distance to uniformity. We investigate connections between design for integration of f with respect to a measure µ (quadrature design), construction of the (continuous) BLUE for the location model, and minimization of energy (kernel discrepancy) for signed measures. Integrally strictly positive definite kernels define strictly convex energy functionals, with an equivalence between the notions of potential and directional derivative showing the strong relation between discrepancy minimization and more traditional design of optimal experiments. Kernel herding algorithms, which are special instances of vertex-direction methods used in optimal design, can be applied to the construction of point sequences with suitable space-filling properties. Several illustrative examples are presented.
En ligne / onlineA standard objective in computer experiments is to predict/interpolate the behaviour of an unknown function f on a compact domain from a few evaluations inside the domain. When little is known about the function, space-filling design is advisable: typically, points of evaluation spread out across the available space are obtained by minimizing a geometrical (for instance, minimax-distance) or a discrepancy criterion measuring distance to uniformity.
We focus our attention to sequential constructions where design points are added one at a time. The presentation is based on the survey], built on several recent results that show how energy functionals can be used to measure distance to uniformity. We investigate connections between design for integration of f with respect to a measure µ (quadrature design), construction of the (continuous) BLUE for the location model, and minimization of energy (kernel discrepancy) for signed measures. Integrally strictly positive definite kernels define strictly convex energy functionals, with an equivalence between the notions of potential and directional derivative showing the strong relation between discrepancy minimization and more traditional design of optimal experiments. Kernel herding algorithms, which are special instances of vertex-direction methods used in optimal design, can be applied to the construction of point sequences with suitable space-filling properties. Several illustrative examples are presented.