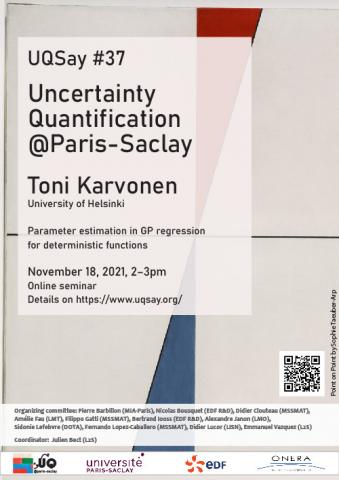
UQSay Seminar #37: Parameter estimation in GP regression for deterministic functions
In fields such as kriging, modelling of computer experiments, and probabilistic numerical computation, Gaussian process (GP) regression is used to interpolate deterministic functions which are observed without noise on compact sets. This talk reviews recent theoretical work on estimation of parameters (in particular via maximum likelihood) of the covariance kernel of the GP prior in such a setting, as well as the effect parameter estimation has on uncertainty quantification under model misspecification. We also discuss results on sample path properties of GPs that we use to characterise data-generating functions which resemble samples from a GP and to highlight the difference in assuming that the data are generated by some deterministic function or by a stochastic process. The results are based on the theory of reproducing kernel Hilbert spaces and function approximation in Sobolev spaces, which are briefly reviewed.
En ligne / onlineIn fields such as kriging, modelling of computer experiments, and probabilistic numerical computation, Gaussian process (GP) regression is used to interpolate deterministic functions which are observed without noise on compact sets. This talk reviews recent theoretical work on estimation of parameters (in particular via maximum likelihood) of the covariance kernel of the GP prior in such a setting, as well as the effect parameter estimation has on uncertainty quantification under model misspecification. We also discuss results on sample path properties of GPs that we use to characterise data-generating functions which resemble samples from a GP and to highlight the difference in assuming that the data are generated by some deterministic function or by a stochastic process. The results are based on the theory of reproducing kernel Hilbert spaces and function approximation in Sobolev spaces, which are briefly reviewed.