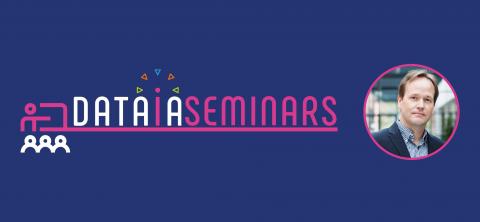
DATAIA Seminar | « Linear pooling of sample covariance matrices » - Esa Ollila
Esa Ollila (Université de Aalto) est l’animateur du séminaire DATAIA du mois de décembre sur le thème « Linear pooling of sample covariance matrices ».
Résumé :
We address the problem of estimating high-dimensional covariance matrices of multiple classes (populations) in the small sample size setting. We propose to estimate each class covariance matrix as a weighted linear combination of sample covariance matrices of individual classes. The optimal weights that yield the minimum mean squared error (MSE) are then derived. The proposed estimator based on optimal weights is shown to attain significant reduction in estimation error when sample sizes are limited and/or when at least some of the true class covariance matrices share similar structure. We develop an effective method for estimating the optimal weighs in the case that the true unknown populations are members of elliptically symmetric distributions with finite fourth-order moments. To this end, we utilize the spatial sign covariance matrix, which we show (under rather general conditions) to be an unbiased estimator of the normalized covariance matrix as the dimension grow to infinity. We also show how the proposed approach can be used in choosing the regularization parameters for multiple target matrices in a single class covariance matrix estimation problem. Our approach is general, and is applicable also for complex-valued data. We illustrate the effectiveness of the proposed covariance matrix estimators via numerical simulation studies and portfolio optimization problem using real historical stock data wherein the proposed approach is able to outperform several state-of-the-art methods. Finally, an application in radar signal processing of complex-valued data is also discussed. This is a joint work with Elias Raninen (Aalto University) and David E. Tyler (Rutgers University, USA)
Esa Ollila (Université de Aalto) est l’animateur du séminaire DATAIA du mois de décembre sur le thème « Linear pooling of sample covariance matrices ».
Résumé :
We address the problem of estimating high-dimensional covariance matrices of multiple classes (populations) in the small sample size setting. We propose to estimate each class covariance matrix as a weighted linear combination of sample covariance matrices of individual classes. The optimal weights that yield the minimum mean squared error (MSE) are then derived. The proposed estimator based on optimal weights is shown to attain significant reduction in estimation error when sample sizes are limited and/or when at least some of the true class covariance matrices share similar structure. We develop an effective method for estimating the optimal weighs in the case that the true unknown populations are members of elliptically symmetric distributions with finite fourth-order moments. To this end, we utilize the spatial sign covariance matrix, which we show (under rather general conditions) to be an unbiased estimator of the normalized covariance matrix as the dimension grow to infinity. We also show how the proposed approach can be used in choosing the regularization parameters for multiple target matrices in a single class covariance matrix estimation problem. Our approach is general, and is applicable also for complex-valued data. We illustrate the effectiveness of the proposed covariance matrix estimators via numerical simulation studies and portfolio optimization problem using real historical stock data wherein the proposed approach is able to outperform several state-of-the-art methods. Finally, an application in radar signal processing of complex-valued data is also discussed. This is a joint work with Elias Raninen (Aalto University) and David E. Tyler (Rutgers University, USA)