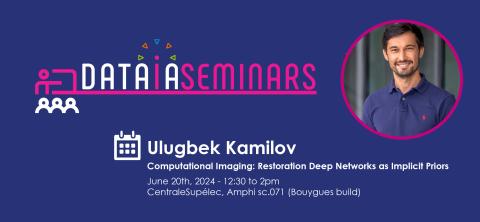
Séminaire DATAIA | Ulugbek Kamilov
Titre
"Computational Imaging: Restoration Deep Networks as Implicit Priors"
Résumé
Many interesting computational imaging problems can be formulated as imaging inverse problems. Since these problems are often ill-posed, one needs to integrate all the available prior knowledge for obtaining high-quality solutions. This talk will explore a series of techniques that leverage deep neural networks for image restoration as data-driven, implicit priors for images. The methods discussed originate from the well-known plug-and-play (PnP) methodology, known for its effectiveness in addressing imaging inverse problems. We will extend the conversation to the generalization of PnP methods, moving beyond traditional use of additive white Gaussian noise (AWGN) denoisers to include a variety of other restoration networks. This expansion not only enhances imaging performance but also offers the flexibility to train priors in the absence of clean data. Additionally, the talk will cover the theoretical underpinnings of using deep restoration networks and their applications in biomedical image reconstruction.
Titre
"Computational Imaging: Restoration Deep Networks as Implicit Priors"
Résumé
Many interesting computational imaging problems can be formulated as imaging inverse problems. Since these problems are often ill-posed, one needs to integrate all the available prior knowledge for obtaining high-quality solutions. This talk will explore a series of techniques that leverage deep neural networks for image restoration as data-driven, implicit priors for images. The methods discussed originate from the well-known plug-and-play (PnP) methodology, known for its effectiveness in addressing imaging inverse problems. We will extend the conversation to the generalization of PnP methods, moving beyond traditional use of additive white Gaussian noise (AWGN) denoisers to include a variety of other restoration networks. This expansion not only enhances imaging performance but also offers the flexibility to train priors in the absence of clean data. Additionally, the talk will cover the theoretical underpinnings of using deep restoration networks and their applications in biomedical image reconstruction.